
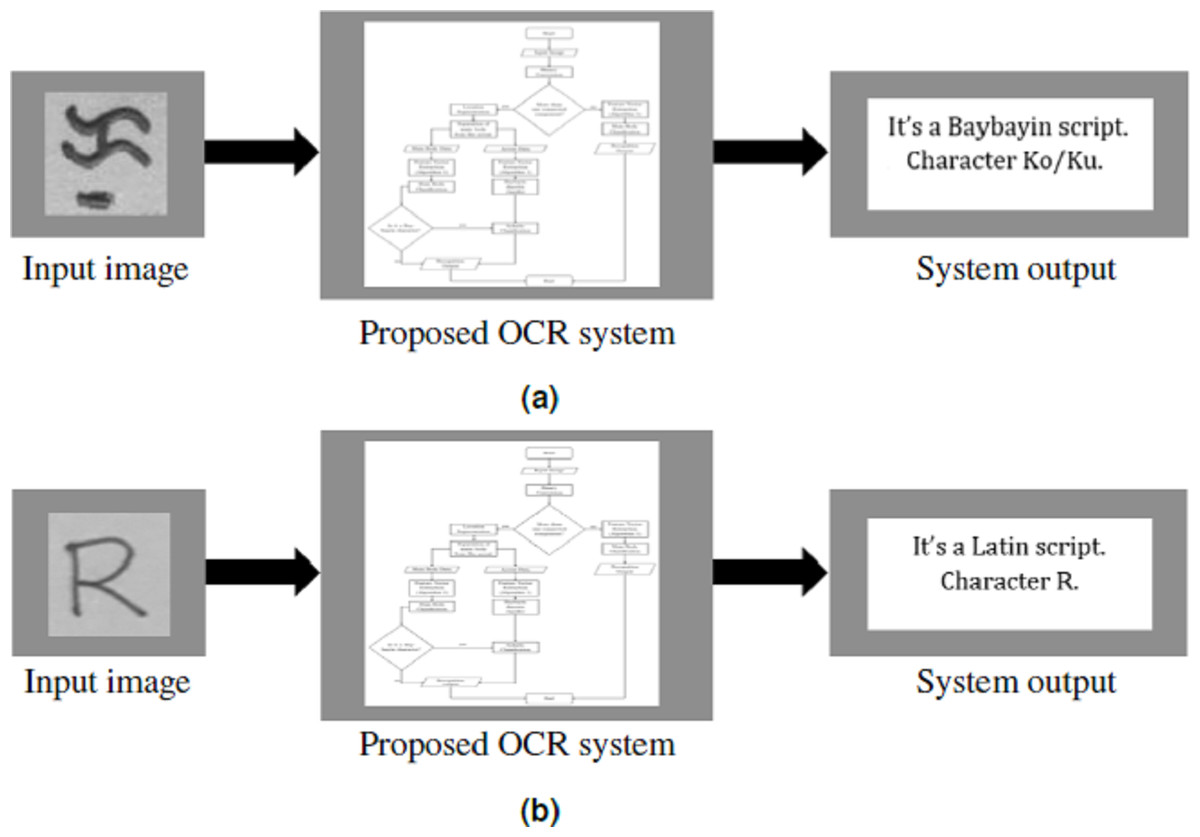
3 illustrates a block diagram of full architecture. The final output shape must be 25 × 72 to fit in the CTC loss function. The bidirectional RNN layer’s output passes through a fully connected dense layer with a softmax activation function and provides the final output. We experiment with these two modified versions of RNN and evaluate their accuracy in the results analysis section. Since general RNN has vanishing gradient problems, we use two modified RNN, Long Short Term Memory(LSTM) Īnd Gated Recurrent Unit (GRU) to avoid vanishing gradient problems. In the bidirectional RNN model, two bidirectional RNN layers are assigned to predict the word from the image by analysing the features’ sequence. The features obtained from the baseline model is passed to the bidirectional RNN model. Therefore, we experiment with four different convolutional architectures and evaluate their performance in the results analysis section. To build the end to end system, we fix operational output as 25 × 72 Convolutional layers take input images, extract useful features and give a lower-dimensional output. We have modified the pre-trained convolutional model by deducting some layers, guaranteeing 25 left-to-right frames for the end-to-end method.

To create the baseline model, we use famous CNN architectures rather than building our own to minimise the workload and get the full feature. Then, the baseline model directly receives input images and extracts high dimensional features. The input layer passes the image in the shape of 200 × 50 × 3 to get the best results in our architecture. The feature extraction process is segmented into two parts in our architecture: a baseline model and a stack of bidirectional RNN model. Therefore, many researchers have made efforts to transform a document into a machine-readable file since the middle 1950s.įeature extraction is the process of finding necessary information from an image to identify the word. Implementing an OCR system is not easy as machines cannot perceive information from an image like the human brain. Also, typewritten recognition systems’ success rate is more than the handwritten ones, as they are less complicated and less variation is observed. Typewritten OCR systems are more likely easy to implement than handwritten OCR systems.
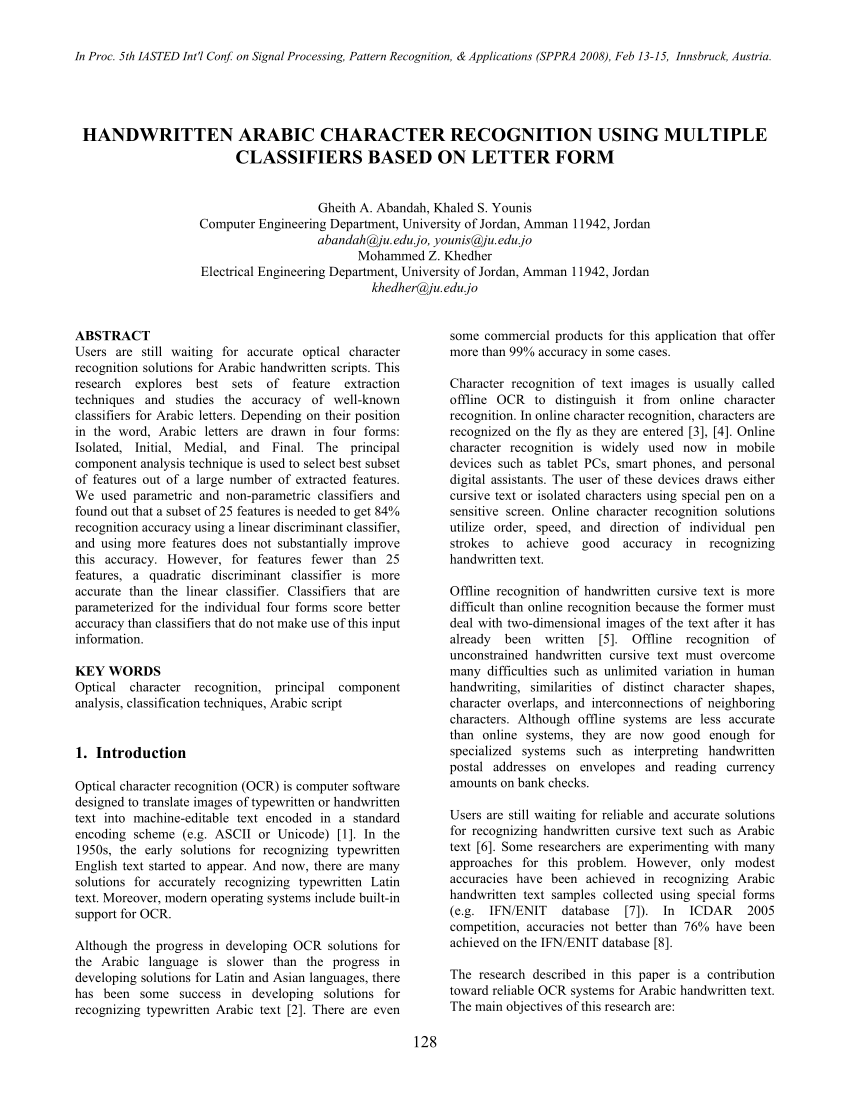
On the other hand, handwritten scripts are written by humans and then recognised by the OCR system. Typewritten scripts typed in computers before the process of recognition starts. The OCR system has two major categories: typewritten and handwritten scripts. With the development of digital computers and scanning devices, OCR technology is improved in the middle of the 1950s. OCR systems can benefit organisations by facilitating with better process speed, enhanced workforce, and lower costs. People can use an OCR to reduce the complexity of digitising documents manually.
#HANDWRITTEN JAPANESE OCR LICENSE#
OCR is used in diverse domains including banks, post-offices, defence organisations, license plate recognition, reading aid for the blind, library automation, language processing, multimedia system design, education institute, etc. OCR systems are used for converting books, documents, and images into a computerised file. It has a broad area of research due to its usefulness. There are different types of OCR systems, including intelligent word recognition, intelligent character recognition, optical word recognition, optical character recognition, and optical mark recognition. OCR is a popular technology used in automated data capture solutions and document classifications.
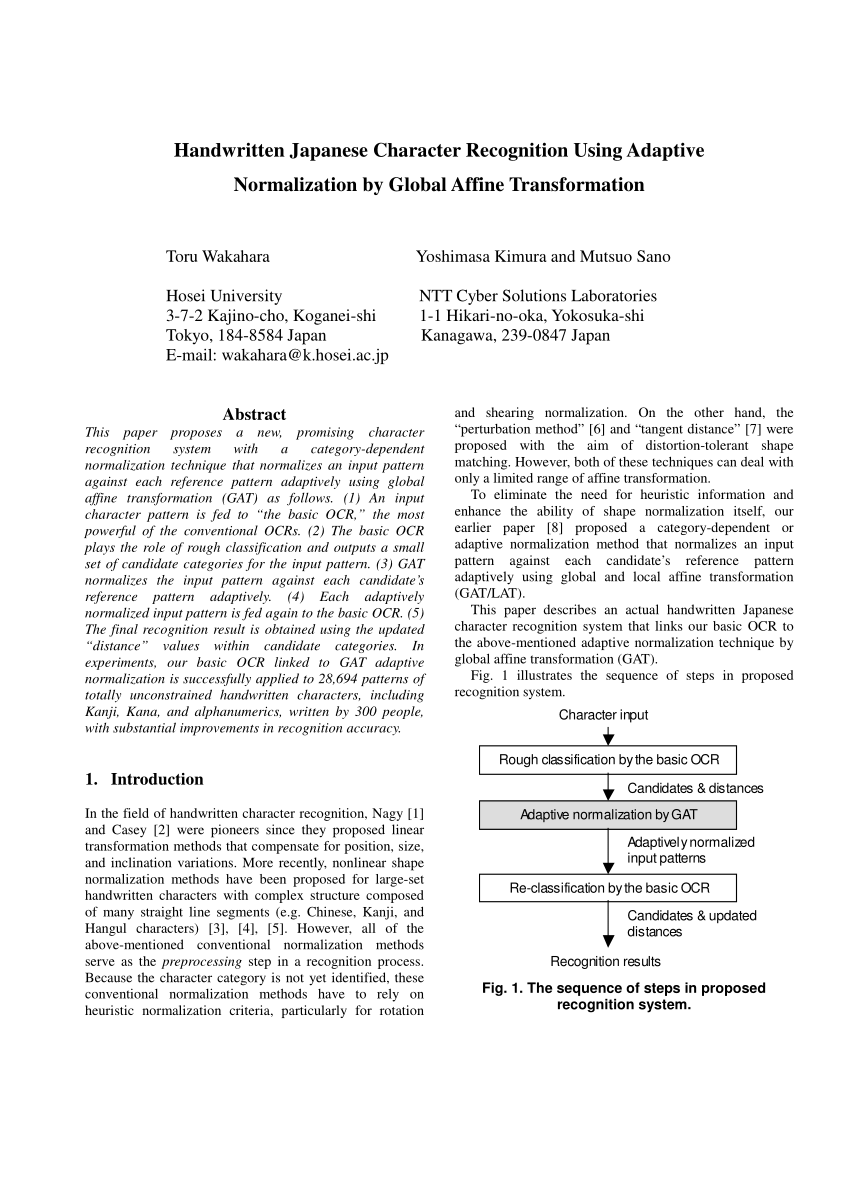
OCR scans an image and generates a machine-encoded file. The proposed method achieves 0.091 character error rate andĠ.273 word error rate performed using DenseNet121 model with GRU recurrent Using BanglaWritting dataset, which is a peer-reviewed Bengali handwritten Further, we experiment with two different recurrent neural Including DenseNet, Xception, NASNet, and MobileNet to build the OCRĪrchitecture. WeĮxperiment with popular convolutional neural network (CNN) architectures, That recognises handwritten Bengali words from handwritten word images. The proposed architecture implements an end to end strategy This paper introduces an end-to-end OCR system forīengali language. Among them, most of the works focused on OCR of Despite this, very few works are available in case of Non-commercial OCR systems exist for both handwritten and printed copies forĭifferent languages. Optical character recognition (OCR) is a process of converting analogueĭocuments into digital using document images. End-to-End Optical Character Recognition for Bengali Handwritten Words
